Essential Number of Principal Components and Nearly Training-Free Model for Spectral Analysis
Source Code
Zip File : https://m-07225.ad336.08cc.data.globus.org/home/lipitao/FPCA_code.zip
—————————————————————————————————————————
Multi-Label Classification with Optimal Thresholding for Multi-Composition Spectroscopic Analysis
In this paper, we implement multi-label neural networks with optimal thresholding to identify gas species among a multiple gas mixture in a cluttered environment. Using infrared absorption spectroscopy and tested on synthesized spectral datasets, our approach outperforms conventional binary relevance-partial least squares discriminant analysis when the signal-to-noise ratio and training sample size are sufficient.
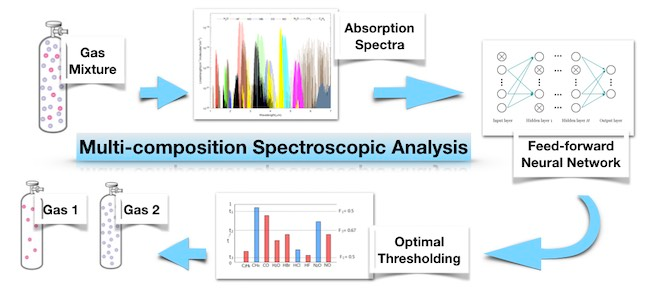
Published Papers
- Yifeng Bie, Shuai You, Xinrui Li, Xuekui Zhang, Tao Lu, “Essential Number of Principal Components and Nearly Training-Free Model for Spectral Analysis”
- Luyun Gan, “Multi-label classification with optimal thresholding for multi-composition spectroscopic analysis,” UVicSpace, ETD (Electronic Theses and Dissertations)
- Luyun Gan, Brosnan Yuen and Tao Lu, “Multi-Label Classification with Optimal Thresholding for Multi-Composition Spectroscopic Analysis,” Mach. Learn. Knowl. Extr. 2019, 1(4), 1084-1099